Protein research has relied for many years on the availability of structure information and evolutionary similarity. Although significant advancements have been achieved, current research still focuses either on generalised research models or already studied proteins. We know that the protein landscape is immense. Metagenomic experiments suggest that 365,000,000+ unique protein sequences identified in nature are just a tip of the iceberg. Thus, there is still virtually unlimited potential to be uncovered and discovered. Novel AI language model approaches now bring an opportunity to study proteins in an unbiased manner.
Transformers: Innovative language models applied to Biology
Powered by InstaDeep, DeepChain™ has been launched as an AI-powered protein exploration and design platform with its Playground module leveraging the power of transformer algorithms as an unbiased way to uncover novel protein sequences and discover new protein properties.
Transformers are language models that can be trained to understand and process language. When queried on a sentence, the model provides the probability of observing its words in that particular order taking into consideration the rest of the sentence. Importantly, transformers are not limited to the database of sentences they are trained on, but they rather learn patterns and can come up with new combinations of words, offering great potential for exploration of the language of interest.
For their use in biology, transformers are trained on the language of proteins, understanding protein sequences as sentences and amino acids as words. In our case, the transformer on DeepChain’s Playground module has been trained with UniRef100 database, which comprises all non-redundant protein sequences known to humanity, and can provide numerous interesting insights to empower your protein research efforts.
In this blog, we are going to show how you can use the freely accessible Playground module to identify the key positions in a protein sequence, due either to functional or structural evolutionary constraints, to inform your research and exploration of protein variants.
Gain insights on what are the key amino acids and where these locate within the 3D structure of your protein of interest
As an example, let us look at the human ubiquitin. Ubiquitin is a small protein, only 76 amino acids long. It is highly evolutionarily conserved, present in all eukaryotic cells. Ubiquitin binds substrate proteins to regulate their function and it is implicated in crucial cellular functions such as protein degradation, programmed cell death (apoptosis), cell cycle, DNA transcription and repair, and signal transduction.
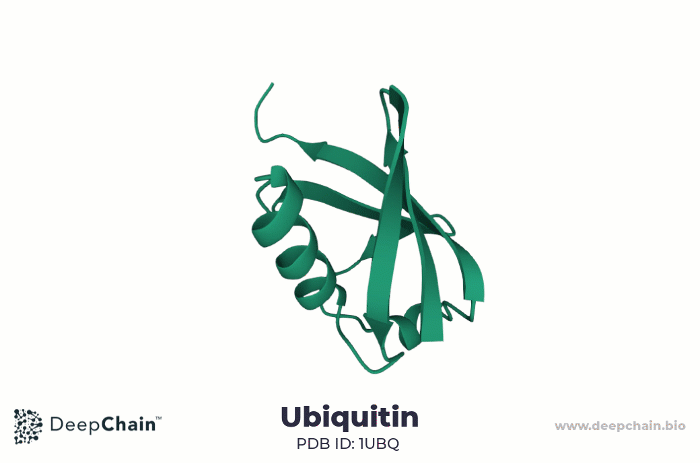
Cartoon representation of ubiquitin’s 3D structure
When we input ubiquitin’s sequence in DeepChain’s Playground module, the model returns a heatmap with an estimated distribution of amino acid probabilities (Y axis) for each position (X axis) of the protein sequence.
We can quickly see that almost all amino acids in ubiquitin have a posterior probability nearing 100%, which means that, from a language perspective, these and only these particular residues make sense in this order. For most proteins, inputting a wild-type sequence will return similar results, since proteins have evolved to their most perfect state as it is the case for ubiquitin, and then these sequences became fixated in evolution, being passed to the offspring and descendant species in virtually unmodified form.
In order to facilitate further protein sequence exploration, we have added the ability to analyse different temperature scenarios. By increasing the temperature, we can make the system permit more diversity by flattening the probability distribution, and thus make it highlight which positions could tolerate a change, given external pressure to mutate.
For the ubiquitin example, by increasing the temperature to a value of 100, we can observe that there are many regions in the sequence that now allow amino acid variation.
Even more interestingly, there are some positions within the sequence where the current amino acids still appear with 100% preference, signaling their nearly unconditional relevance. For the ubiquitin protein, we have plenty of experimental evidence showing why these amino acids are key for the protein. The string of lysines (K) in its sequence is associated to protein function, with lysine 6 thought to be associated with DNA repair, lysine 29 being involved in lysosomal degradation, lysine 48 being essential in protein degradation and lysine 63 being responsible for cellular signaling (ref). Similarly important, the amino acid glutamine (Q) in position 41 has been shown to be needed for structural stability of the protein (ref).
In a completely unbiased manner the transformer in the Playground is capable of understanding which amino acids are key for both function and structure of ubiquitin in a matter of seconds. All of this, without need to conduct homology search, build multiple sequence alignments, or even rely on any external sources of information beyond the sequence itself. This makes it a valuable source of insights to inform protein exploration research and mutation experiments to gain understanding on the protein’s function. However, it can also be leveraged to complement structural and conservation data if available for your protein of interest.
Further to this, if the structure of your protein of interest is available, you can input the PDB file into the Playground to map the information contained in the heatmap onto the 3D protein visualization. This can further facilitate the analysis of these identified key amino acids by understanding where they sit on the structure, which can support the generation of new hypotheses on protein sequence, structure and function.
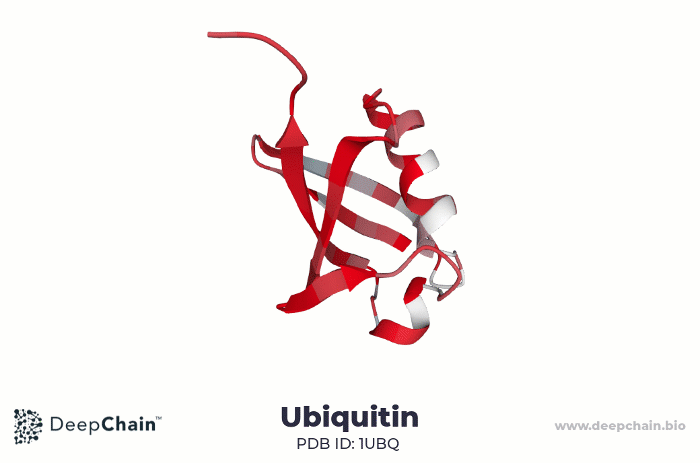
Ubiqutin’s 3D structure overlaid with mutation tolerance information from the transformer. Red regions have low mutation tolerance, whilst light-blue regions have high mutation tolerance
Take it for a spin!
The DeepChain™ Playground module leverages transformer algorithms and is now accessible for free to analyse your protein sequences of interest and to discover variants and key regions. Create your personalised and secure account and start using AI to accelerate and improve your design process leading to key discoveries by registering here.
If you are a computational biologist passionate about AI, please consider joining our team! You can find our job offers for a biology-based position here and here.
To learn more about DeepChain™, feel free to send us an email at hello@deepchain.bio!