We are opening DeepChainTM to allow users to ideate and build their own machine learning (ML) tools, or as we call them, Apps, to solve their particular biology questions. We offer pre-trained models, AI and biology expertise, technical support and the infrastructure needed to maximise your research.
Scientific advancements have allowed the generation of large datasets to inform protein research and scientists have an increasing need to tap into the insights to make faster informed decisions on their work. Gaining access, analysing and processing the learnings derived from big data to answer personal biological questions is highly complex. There is a requirement to combine biology expertise, AI technical skills and efficient computing resources.
Understanding this challenge, DeepChainTM aims to democratise access to AI protein exploration and design tools using our powerful supercomputing infrastructure.
Expanding DeepChainTM to tackle the complexity of biology research
Transformer models, advanced natural language processing AI models, have been a recent development and success across multiple industries. Their power resides on being trained in a self-supervised manner, so that large datasets can be leveraged without the need for manual labeling.
At DeepChainTM we make transformer models such as prot_bert and esm trained on millions of protein sequences from the UniRef100 and Big Fat Databases readily available to the user. Upon training, these models gain an understanding of the language of proteins and are powerful tools to understand protein evolution and sequence variability among others (for more information, read our transformer blogs Transformers: An AI-Language Model Redefining Protein Research and Design and AI-Language Models Offer a Novel Approach to Protein Sequence Exploration).
More importantly, DeepChainTM offers access to these protein embeddings or vector maps generated by the transformer model as a stepping stone for transfer learning and fine-tuning of personalised machine learning models to support biology research. The user can leverage pre-trained DeepChainTM transformers and retrain the model with a labelled dataset that can answer their particular question.
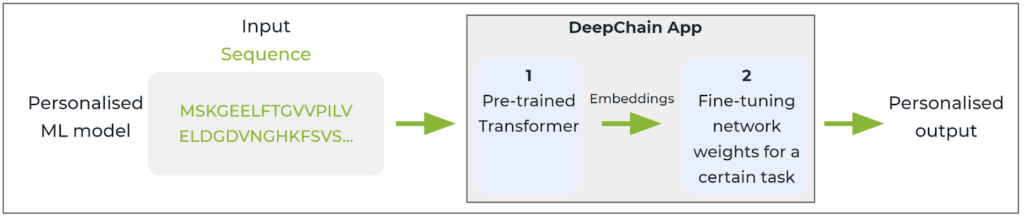
A DeepChainTM App or personalised ML model utilises DeepchainTM protein embeddings to fine-tune the outputs according to the research question of interest.
Users can train ML models that score for any specific characteristic they might be interested in, essentially offering unlimited opportunities for researchers to tap into the latest AI innovations and super computing infrastructure to solve their distinctive research questions. These Apps or scoring systems can be understood as either classifiers or predictors.
- Classifiers to explore proteins and datasets. Train an ML model to identify and classify a particular characteristic. This serves to classify large datasets to group similar proteins and/or analyse individual proteins to understand the category they fit in.
- Predictors to design new proteins or analyse known variants. Train an ML model to score for a particular characteristic. This offers a method to design new protein sequences maximising or minimising for this score. Predictors can also be used to understand how a protein variant differs from the standard sequence according to a set score.
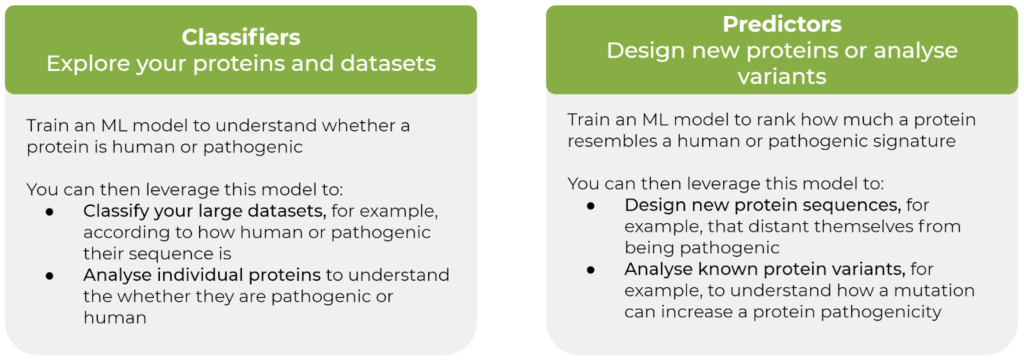
Use cases for an App or ML model trained to distinguish between pathogenic and human sequences.
Using pre-trained models from the DeepChainTM platform has several benefits over training ML models from scratch, including time-saving, increased accuracy and the ability to use smaller labelled datasets.
Example: DeepChainTM App for classifying human vs pathogen sequences
As an example of a research question, we want to detect and classify human proteins from pathogen proteins based on sequence alone. The user will leverage DeepChainTM pre trained models and will retrain the model with a set of supervised data related to this particular research interest. In this case, we will leverage a dataset of ~96,000 protein sequences that has appropriate labelling and distribution for the question at hand, with 50% human and 50% pathogen sequences.
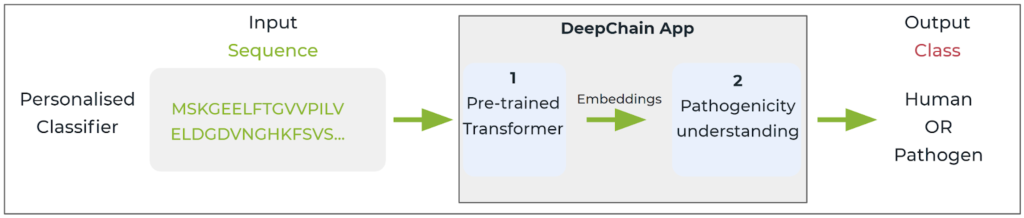
Workflow of a personalised DeepChainTM App trained to categorise sequences as human or pathogen.
The training is conducted on DeepChainTM Jupyter Notebooks and the model is ready in just a day. With this model, the DeepChainTM team managed to obtain a validation area under curve (AUC) score of 99.9%.
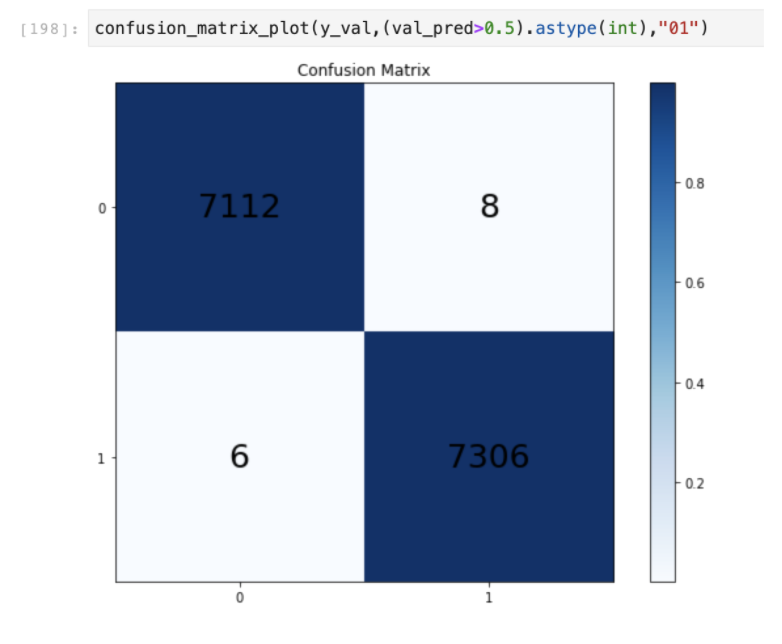
Confusion matrix for the test set. All the entries in the diagonal are correctly classified. Out of the 14,000 samples only 16 were incorrectly classified.
Learn more about it
Karim Beguir, Co-Founder & CEO at InstaDeep, and Nicolas Lopez Carranza, DeepChainTM Product Lead, presented the possibilities of a practical approach to leveraging DeepChain’sTM unparalleled capabilities for building personalised scorers at NVIDIA’s GTC21 event. Watch the full presentation here.
Want to get involved?
Visit DeepChain’s™ GitHub to learn more about the technical aspects of building ML models and engage with our community of experts.
The DeepChain™ Playground module leverages transformer algorithms and is accessible for free to analyse your protein sequences of interest and to discover variants and key regions. Create your account here and start using AI to accelerate and improve your design process leading to key discoveries.
To learn more about DeepChain™, feel free to send us an email at hello@deepchain.bio!If you are a computational biologist passionate about AI, join our team! You can find our job vacancies here.